Regulating data-powered artificial intelligence
Published on 14th Jun 2022
Artificial intelligence (AI) is an increasingly powerful and pervasive tool being used to boost productivity across all sectors. Data is its essential fuel. A wave of new regulation is coming at EU level both for AI specifically and for the data needed to feed it. The latter will boost availability of data, but compliance will need to be built – or retrofitted – into AI tools to avoid the risk of enforcement action and fines.
This is chapter 2.7 of Data-driven business models: The role of legal teams in delivering success
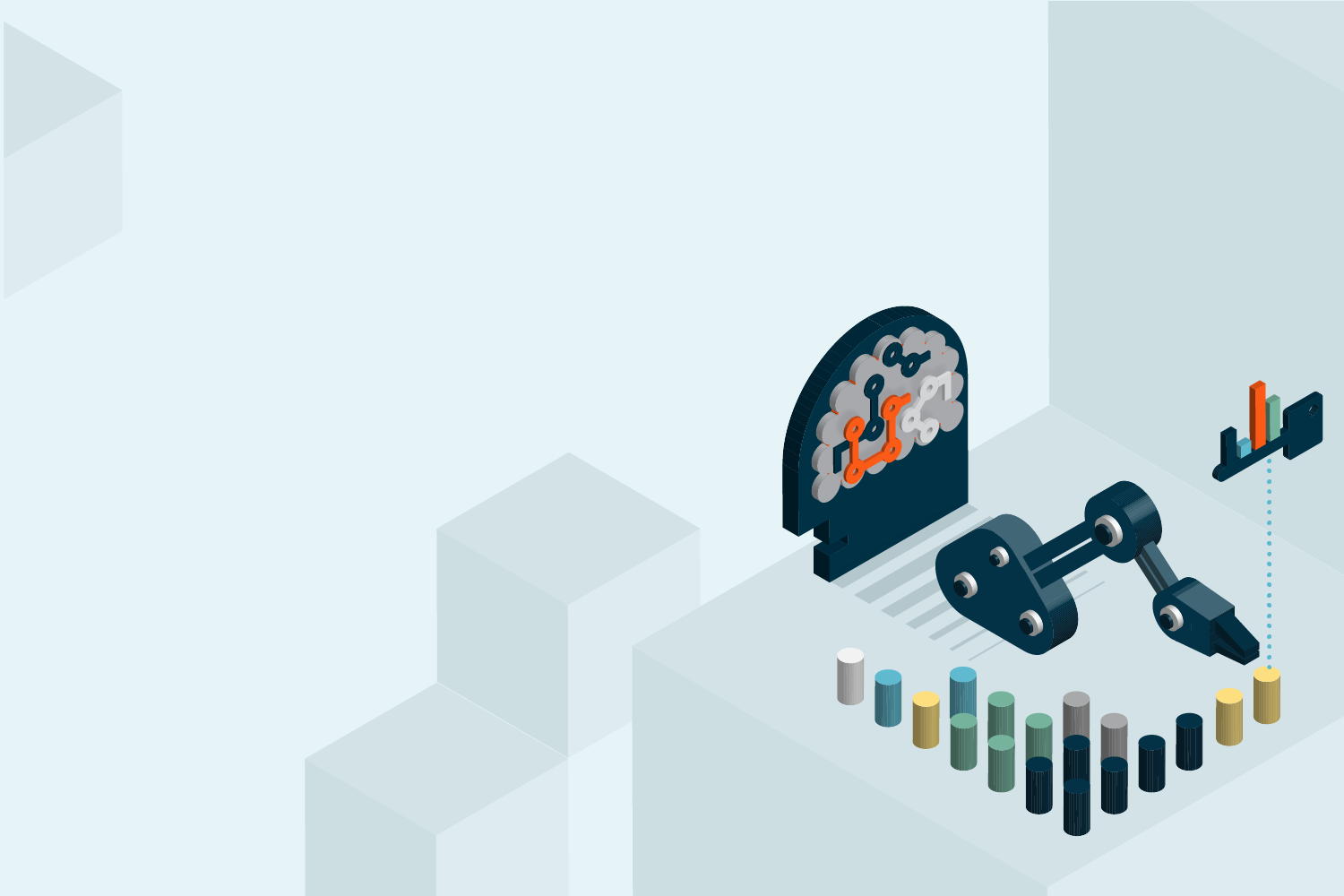
Key Takeaways
|
The importance of data for artificial intelligence systems
AI, in its machine learning and deep learning forms, depends on data.
AI systems are trained to perform their allocated tasks using vast quantities of information. The systems are set up to identify and map patterns in the training data, creating a huge matrix that is subtly recalibrated and refined with each new piece of data that passes through the system. Once trained, the AI system can be used to classify new pieces of data based on the data that it has been trained on, or to generate data that is similar to its training data.
AI is used for a vast range of commercial applications. It can be used for decision-making – this information matches that grouping and so produces that outcome; this person can be classified as low risk so can be given consent for a new credit card; this candidate matches the preferred characteristics so should be interviewed; that customer query corresponds to this answer. It can also be used for interpretation of visual images or text – this image is a dog; that scan has cancerous cells; this sentence in one language translates into that sentence in another; there is a person on that crossing. It can also be used for prediction tasks – this drug may match that medical problem; this new text answers that question; this machine part is likely to break down around that date; these related website links may be useful for someone reading that webpage.
The applications that AI can be put to are hugely varied, but each individual tool has only 'narrow' intelligence, in the sense that it is trained for a very specific task and objective. Although AI systems can sometimes find patterns in data that humans had not spotted, and so generate outputs that humans did not expect, they are entirely constrained by the scope of the data that has been passed through them. A system for recognising apples that has not been shown enough pears or oranges would not be able to distinguish between them. AI systems cannot map ideas, concepts or data that they have not been shown through the training datasets, and have no wider common sense or understanding of the context in which they are deployed.
Accordingly, AI systems can only reflect and replicate the data passed through them. If the training data is poor quality, unrepresentative or biased, then the resulting AI will very likely reproduce those problems and generate outputs that are poor quality, unrepresentative or biased. As the data scientists put it, "Garbage in, garbage out".
For all these reasons, the availability of data, its quality, and its appropriateness for the task in hand, are all key considerations when developing and using AI tools. Given the breadth of applications of AI, poor decision-making could clearly generate harm to businesses or individuals in a myriad of ways.
![]() Data-driven business modelsThe role of legal teams in delivering success |
Regulation to ensure trustworthy AI
The proposed AI Act
In April 2021, the European Commission published proposals for a new cross-sector regulatory regime for AI. The legislation takes a risk-based approach:
- AI tools in a limited number of defined areas (such as social scoring or real time facial recognition surveillance systems in public areas for law enforcement) will be prohibited, subject to exceptions;
- AI systems considered to be "high risk" will be subject to extensive regulation with an enforcement framework at national level that includes the potential for heavy GDPR-style fines for non-compliance;
- AI systems designed to interact with humans will be subject to transparency requirements to make sure the person concerned knows they are dealing with an AI tool, not a human; and
- Other AI applications are left unregulated.
Broadly speaking, the "high risk" categories are focused on AI tools used in health and safety systems, or which impact on fundamental human rights – in both cases the focus is on the risk of harm to natural persons. For example, the proposed high risk categories include biometric ID systems, credit scoring systems, a number of HR systems such as job application sifting, work allocation or employee performance measuring, as well as AI systems used in the public sector such as social security assessments, border control checks or asylum eligibility systems. The draft recitals highlight
the risk that AI systems could perpetuate historical patterns of discrimination, create new discriminatory impacts, or result in injustices against individuals.
National regulators will be created (or powers extended) to ensure enforcement of the new regulatory framework. Businesses will be required to undertake conformity assessments and in most cases will be able to self-certify compliance. AI tools in conformity will carry the CE mark, and must be registered on a central register maintained by the Commission. In addition to the data provisions discussed below, high risk AI must also meet mandatory requirements in relation to technical documentation, record-keeping, wider transparency issues, human oversight, plus accuracy, robustness, and cybersecurity.
The EU's approach can be seen as another example of its strategic ambition to set the gold standard for digital regulation around the world. It is certainly the most advanced legislative proposal in this field and is ambitious in its aim to create a single horizontal regime, rather than more tailored regulation for different sectors or risk areas.
The proposals regarding data
Since data is the fuel for AI, the provisions regarding data are likely to be correspondingly significant in their impact. Obligations for high risk AI systems concerning data and data governance training are addressed in Article 10 of the proposed regulation. The proposals are demanding and extensive:
- Data governance and management practices must cover design choices; data collection; relevant data preparation processes; the assumptions about what the data measures and represents; prior assessment of the availability, quantity, and suitability of the required datasets; examination for possible bias; and the identification of possible gaps or shortcomings in the data and how those gaps and shortcomings can be addressed.
- Datasets must be relevant, representative, free of errors, and complete, and must have appropriate statistical properties for the intended application of the AI tool concerned.
- Where appropriate, datasets must take into account the particular characteristics or elements of the specific geographical, behavioural, or functional setting in which the high-risk AI system will be deployed.
The standard required of data is important because this is one of the limited areas where the highest level of fines envisaged under the proposals for non-compliance – up to six per cent of global turnover – will apply.
The intention of these provisions is to ensure that datasets are properly and responsibly curated to minimise the risk of poor quality data resulting in a poor quality tool more likely to cause harm. But the final provisions are likely to incorporate amendments. The Commission's proposals are demanding to a point that many commentators consider both unrealistic and disproportionate, requiring a near-perfect standard of data, which may simply not be achievable. Given the severity of the potential fines, it is important that compliance does not involve disproportionate difficulty or burdens on businesses.
Opening up access to data
As well as quality of data, access to data is essential for a thriving AI ecosystem. The AI Act does not address this issue, but it is one of the focuses of separate legislative initiatives at EU level, flowing from the European Data Strategy.
The Commission's November 2020 proposal for a Data Governance Act seeks to shape the data ecosystem by encouraging the availability of public sector data, by regulating for-profit intermediaries that supply data to optimise consumer trust, and by providing for new data sharing structures for individuals to "donate" their data to not-for-profit organisations that will be able to supply data for defined purposes. The Data Governance Act is discussed in Chapter 2.12.
Separately, the Commission's proposed Data Act of February 2022 makes provision for opening up access to privately held datasets, and for enhanced portability of data. The Data Act is discussed further in Chapter 2.10.
The Commission is also seeking to develop "European Data Spaces", with pools of data for particular sectors, including healthcare and energy.
These initiatives are intended to ensure the availability of data across a wide range of areas to fuel innovation and advances – including developing new AI tools, or improving or updating existing ones.
UK plans to regulate AI
Post-Brexit, the EU legislative initiatives discussed above will not apply directly in the UK (although they will, of course, affect UK and other third country businesses that sell to EU customers).
The UK is not currently planning to take a similar horizontal, one-size-fits-all approach to regulating AI. It is moving somewhat slower than the EU in developing its policy. A White Paper and consultation on how to approach the UK regulation of AI is expected during the course of 2022 (delayed from the first quarter, which the UK's National AI Strategy3 had signalled). Although the UK had previously indicated that a "vertical", sector-specific approach to AI regulation was preferred with policy development led by sector regulators, the White Paper is expected to reopen that decision and seek views through consultation on whether a horizontal approach would be desirable, particularly to ensure conformity of approach between sectors.
Be ready for increasing regulation of AI
Currently, these new areas of legislation are still making their way through the legislative process. Once finalised, each will incorporate a time period for transition before compliance is required. Change is not imminent – but there is no doubt that it is coming.
The regulation of both AI tools and the data that shape them will significantly impact on AI developers, suppliers and organisations using AI. Each of these legislative initiatives from the EU is novel, ground-breaking, and extends digital regulation into new areas where legal compliance had previously been much more limited.
As with so much digital technology, compliance by design will be extremely important. AI-centric business models that do not yet incorporate regulatory compliance – in both product design and data procurement and curation – may require disruptive and potentially costly rethinking. Although the new EU regulations are not yet in force, their future scope and impact needs to be monitored by all those operating and developing AI-centric data-driven business models.