A digital and transformed future | Artificial intelligence supercharging other technology
Published on 8th January 2020
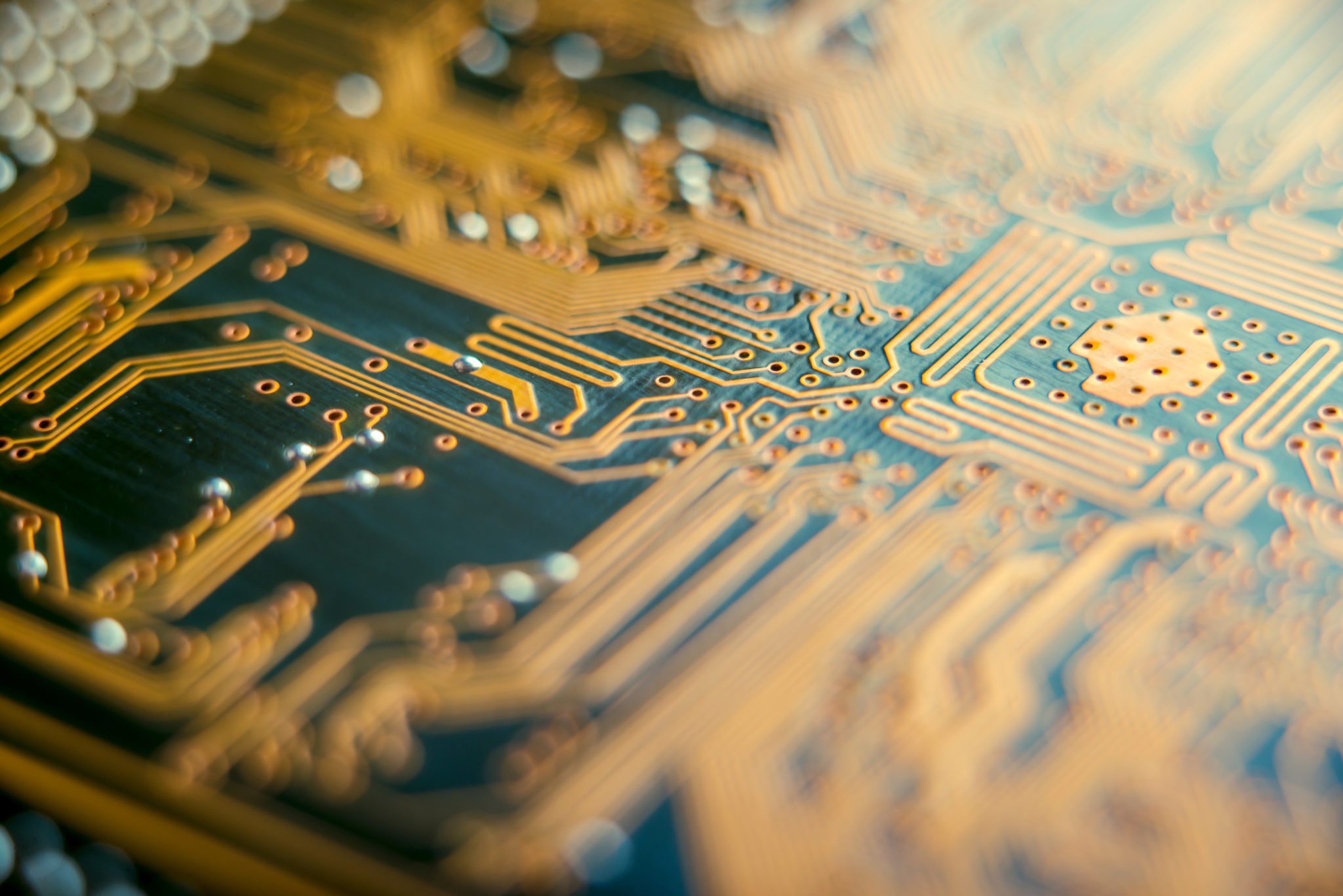
Transformative technology can be powerful not just in its own right, but where different technologies converge. Artificial intelligence, in particular, can be a technology supercharger. The second Insight in our series looking at the digital future (and adapted from an article written for the 2019 Bristol Technology Showcase) considers the transformative power of machine learning.
Artificial intelligence, in the form of machine learning or deep learning, relies on finding and mapping the patterns in data and then using more and more data to refine and deepen the accuracy of that model, without the need for human-generated linear hand-coding.
Part of the reason why this has become such a powerful tool is the speed and availability of almost limitless computing power, thanks to Moore's law and the development of the cloud, respectively. By way of illustration of the current scale, availability and low cost of processing power, a group of computer scientists recently challenged themselves to break the World War II Enigma code using 21st century artificial intelligence. The point of interest is not that they succeeded, but that it took a mere 19 minutes to do so. It might have taken two weeks, but they hired 1000 servers for an hour at a cost of $7.
AI-driven generative design…
A further example of the transformative power of AI is generative design. The design of pieces of kit, such as a bracket for interconnecting different parts or a structural panel in a vehicle, is being optimised using AI. Parameters concerning the structural properties of the piece can be set by the design engineers (for example, the required strength, tolerances, points of connection, areas of open space). The system will then devise numerous potential designs for the piece. To the human eye, generative design pieces often look almost other-worldly because they are so different to what a human mind might design.
The generative design tool can be configured to optimise different design characteristics. A particularly impactful application is to optimise for low weight. This is particularly significant for electric vehicle and aviation design: lower weight reduces the engine power necessary to move the vehicle or aircraft, making it more efficient.
…plus additive manufacturing…
Generative design is used in conjunction with additive manufacturing (a form of industrial-scale 3D printing), which makes it possible to produce these extraordinary new designs. The machines do not need physical retooling to switch to a new design, just a new digital file to drive the output. Small production runs are therefore viable, although additive manufacturing is also being used at scale. Moreover, there are material benefits from additive manufacturing's ability to produce complex shapes in a single piece. Fewer joints makes the piece structurally stronger, more durable and at a lower risk of fracturing, all of which reduces the frequency of repairs.
…plus image recognition-based QA checks
AI can also be used to train systems to recognise faults and errors in the layers of additive manufacturing. Normally, each layer of a 3D-printed product will be photographed as it is printed, and the photographs then subsequently reviewed for quality assurance. An AI image recognition tool, by contrast, can be trained to perform the QA checks and review for errors in real time as the printing machine builds up the layers. The printing process can be stopped if a fatal error is detected, reducing waste by not finishing a faulty product.
Letting robots find their own way
AI has also been used to boost physical robotics. Images of humanoid robots doing backflips or of headless quadruped robots opening doors are immensely impressive. These systems are hand coded, line by line, and take a great deal of time to program, which computing power does not, in itself, make faster. However, the ability of machine learning systems to meet a defined goal from scratch and without linear coding, is now being applied to robotics and is enabling machines to develop the coding needed for a particular task without human input, essentially by trial and error.
Machine learning has been used to work out how to use a robotic hand to manipulate a cube so that a particular face of the cube was selected. A digital model of the hand and cube was created, replicating in virtual form the characteristics and constraints of the physical robot hand and of the cube. The system was given definitions of success and of failure. It then tried the task repeatedly over a period of time until it succeeded in controlling the movements of the fingers and palm sufficiently to manipulate the required face of the cube into the required position.
The coding for the virtual hand was then transferred to control the physical version and the physical hand was able to manipulate the physical cube as required.
AI has been called "software 2.0" for its ability to write itself in this way. Of course, considerable technical skill is needed for these types of project; machine learning typically has a PhD entry level. But increasingly, ready-made AI tools are available "as a Service", including as one of the options in the portfolios of mix-and-match resources and software offered by many of the major cloud computing vendors.
A significant part of the transformative power of AI is this ability to supercharge other technologies. The development of AI tools that can be used off-the-shelf, without the need for highly specialised skills, will only amplify this effect.
This article is part of a series, "a digital and transformed future". For an overview of the series, click here.